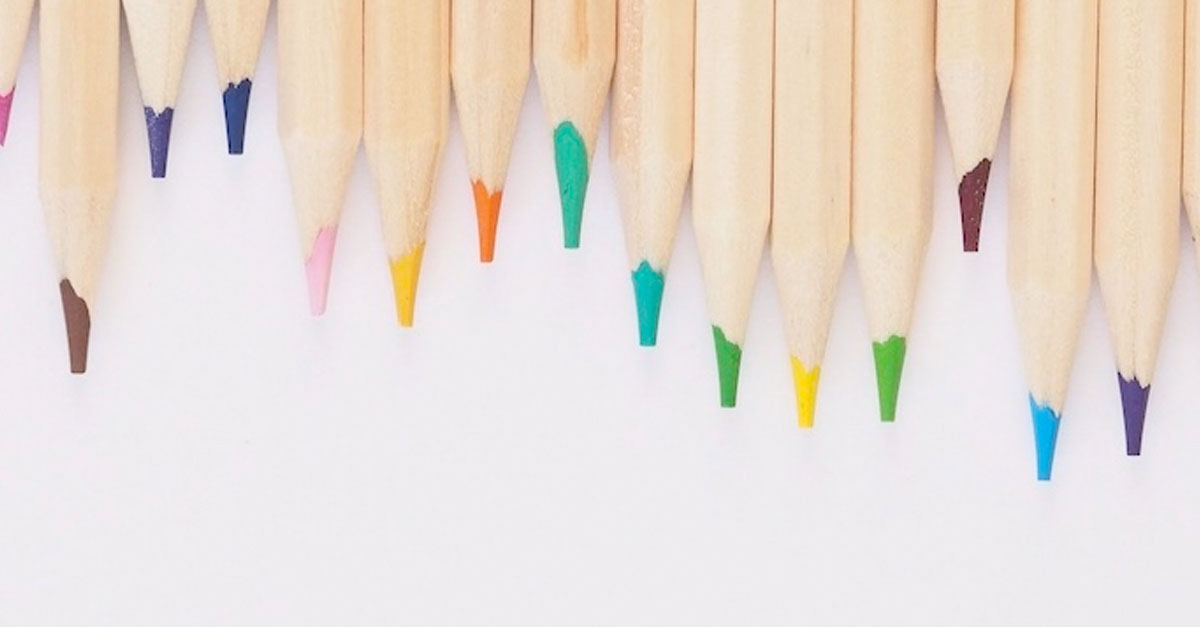
Randomization in Clinical Trials
How can you optimise your participant randomization?
Any reputable research study will need to ensure that effective randomization has taken place so that sound conclusions can be drawn when the study completes. Taking careful consideration over randomization strategies will help to ensure no participant bias and, crucially, avoid unseen or confounding co-factors which might influence your data.
Here's all you need to know about participant randomization techniques.
Simple Randomization : "Round Robin" [1, 2, 1, 2, 1, 2...]
Round robin provides a very simple way of group allocation of participants. Once the number of groups has been defined, participants are allocated in sequence into each group. When participants are allocated once to each group in sequence, the process begins again in the same order.
Pro's of this method
- Very easy to setup.
- An effective way to get equal group sizes.
Con's of this method
- The allocation is very predictable.
- The allocation is not truly random.
Simple Randomization : "Coin Toss" [Heads, Tails, Tails, Heads...]
A very familiar process to most graduates and high school students well-versed in mathematical probability. The coin toss assumes equal bias on each side and, assuming the operator conducts the toss fairly, each new toss will have 50:50 odds.
Pro's of this method
- Very easy to setup.
- No advanced skill or preparation needed.
- Each new toss is independent and not influenced by the previous toss.
Con's of this method
- There can be coin bias / balance risk.
- Requires large numbers to obtain a 50:50 allocation.
- Can only realistically be used to assign 2 groups.
Restricted Randomization : Blocking [ABAB, ABBA, BAAB...]
Blocking is an effective strategy implemented to fulfil the group allocation in an unbiased and unpredictable way. In order to setup blocking, we need to know the group allocation ratio. For example, with two groups which are 1:1, we take those integers representing this ratio and add them together (1 + 1 = 2). The size of the smallest block is the sum of these integers defined from this group allocation ratio. In this scenario our smallest block would be 2. For 3 groups with a group allocation ratio of 1:1:1, our smallest block would be the sum of these integers (1 + 1 + 1 + = 3). This then scales accordingly to however many groups you would require in a study.
Once you have the minimum block size, you can choose to allocate in multiples of this block. For a smallest block of 3, we could use blocks of 3, 6, 9, 12 etc. Once we have the block we wish to use for group allocation, we will then have a list of all possible permutations of this.
In an example of 3 groups (A, B and C), the permutations of random blocks of this would be ABC, ACB, BAC... CBA.
In this scenario, if we had 30 participants in a study, blocking with a block size of 3 would allow for 10 blocks of 3 to provide equal allocation of groups A, B and C. These block allocations are also independent of previous blocks. For example, Block 1: ABC, Block 2: BAC ... Block 10: CBA.
We can represent this in the table example below, and the multinomial coefficient allows us to scale and extrapolate all possible block permutations in any size group.


Pro's of this method
- Ensures consistent group allocation at scale.
- Not predictable.
Con's of this method
- Restrictions on the allocation ratio.
- Restrictions on block size.
- Can help predict future blocks since permutations are known.
Restrictive Randomization: Stratification [Sex, Study Site...]
One of the problems associated with blocking is that additional factors which might later be stratified (for example sex / demographic or study site) to perform sub-group analysis are highly prone to unequal group allocation. In this situation it is prudent to perform stratification of cofactors important in the study design and outcomes before randomization to ensure that these factors are equally assigned.
Pro's of this method
- Ensures cofactors are considered and given equal assignment and weighting.
Con's of this method
- Cofactors which are equally assigned might not actually reflect the target population.
- Unequal assignment might actually be an important consideration itself in the wider context of the research study.
- Too much stratification can lead to imbalances in group allocation.
Adaptive Randomization: "Adapt-as-you-go"
In the case where assignment might need to change according to other factors which are difficult to control for, adaptive randomization is highly useful. For example, if participants have enrolled and produced an equal group allocation, by happenstance, a large number of participants might withdraw from the study before it begins. While we can't easily control for this and must give participants full transparency and options to withdraw, If these are mostly from one group, this can significantly risk the study outcomes. In order to remedy this, an approach like adaptive randomization can be a saving grace. Unequal groups might need 'topping-up' and this method can be used in this case.
Pro's of this method
- Enables the researcher to keep an eye on group allocation and deliberately make groups as equal as possible
Con's of this method
- The randomization is susceptible to human manipulation.
- Can be complicated and require software to assist.
Whatever strategy you decide to implement in your study will need a lot of consideration from your research team. Be sure to take care and use a method which will de-risk the outcomes of your study the most.
Use the contact form here or email us at hello@trialflare.com